Note
Click here to download the full example code
Visualize the input behavior together with the decoded behavior
The goal of this example is to display the input behavior aligned with the decoded behavior in a plot.
By default, this script will download the data to be plotted from AWS S3. If you prefer to use your own data, you can start the closed loop simulation in one terminal:
make run-closed-loop
And then record the streams in another terminal:
recorder --session "decode_example" --lsl "NDS-Behavior,NDS-Decoder" --recording-time 10
Make sure to change the variable:
LOCAL_DATA = True
and replace the variable with the path to your data:
BEHAVIOR_DATA_PATH = "the_path_to_your_recorded_behavior_data.npz"
DECODER_DATA_PATH = "the_path_to_your_recorded_decoder_data.npz"
Environment setup
LOCAL_DATA = False
Set data source
Retrieve the data from AWS S3 or define the path to your local file.
from urllib.parse import urljoin
import pooch
DOWNLOAD_BASE_URL = "https://neural-data-simulator.s3.amazonaws.com/sample_data/v1/"
if not LOCAL_DATA:
BEHAVIOR_DATA_PATH = pooch.retrieve(
url=urljoin(DOWNLOAD_BASE_URL, "decode_example_NDS-Behavior.npz"),
known_hash="md5:201cbd92f432b94ce8dcb8fb87c07634",
)
DECODER_DATA_PATH = pooch.retrieve(
url=urljoin(DOWNLOAD_BASE_URL, "decode_example_NDS-Decoder.npz"),
known_hash="md5:a6a59c08dc32819a6781383e0ba1b4c1",
)
else:
BEHAVIOR_DATA_PATH = "the_path_to_your_recorded_behavior_data.npz"
DECODER_DATA_PATH = "the_path_to_your_recorded_decoder_data.npz"
Load data
Load the data to be plotted.
import matplotlib.pyplot as plt
import numpy as np
from scipy import signal
from sklearn.metrics import r2_score
behavior_file = np.load(BEHAVIOR_DATA_PATH)
behavior_data = behavior_file["data"]
behavior_timestamps = behavior_file["timestamps"] - behavior_file["timestamps"][0]
decoder_file = np.load(DECODER_DATA_PATH)
decoder_data = decoder_file["data"]
decoder_timestamps = decoder_file["timestamps"] - decoder_file["timestamps"][0]
Plot data
def _plot_velocities(
behavior_data: np.ndarray,
behavior_timestamps: np.ndarray,
decoder_data: np.ndarray,
r2: np.ndarray,
axis: int,
):
mean = np.mean(decoder_data[:, axis])
std = np.std(decoder_data[:, axis])
if axis == 0:
plt.title(
(
f"Horizontal direction: r2 = {r2[axis]:.2f}, "
f"mean = {mean:.2f}, std = {std:.2f}"
)
)
else:
plt.title(
(
f"Vertical direction: r2 = {r2[axis]:.2f}, "
f"mean = {mean:.2f}, std = {std:.2f}"
)
)
plt.plot(
behavior_timestamps,
decoder_data[:, axis],
"blue",
label="Decoded (from simulated spikes)",
)
plt.plot(
behavior_timestamps,
behavior_data[:, axis],
"red",
label="Input",
)
plt.ylabel("Velocity (mm/s)")
plt.xlabel("Time (s)")
plt.legend()
def get_lag(x: np.ndarray, y: np.ndarray):
correlation = signal.correlate(x, y, mode="full")
lags = signal.correlation_lags(x.size, y.size, mode="full")
lag = lags[np.argmax(correlation)]
return abs(lag)
h_lag = get_lag(behavior_data[:, 0], decoder_data[:, 0])
behavior_data = behavior_data[:, :]
behavior_timestamps = np.array(behavior_timestamps)[:]
behavior_timestamps = behavior_timestamps - behavior_timestamps[0]
decoder_data = decoder_data[h_lag:, :]
# cut behavior and decoder streams to the same length
min_samples = min(decoder_data.shape[0], behavior_data.shape[0])
decoder_data = decoder_data[:min_samples, :]
behavior_data = behavior_data[:min_samples, :]
behavior_timestamps = behavior_timestamps[:min_samples]
r2 = r2_score(behavior_data, decoder_data, multioutput="raw_values")
dpi = 180
fig_size = (20, 5)
plt.figure(num="Velocities overview", dpi=dpi, figsize=fig_size)
plt.subplot(2, 1, 1)
_plot_velocities(behavior_data, behavior_timestamps, decoder_data, r2, axis=0)
plt.subplot(2, 1, 2)
_plot_velocities(behavior_data, behavior_timestamps, decoder_data, r2, axis=1)
plt.tight_layout()
plt.show()
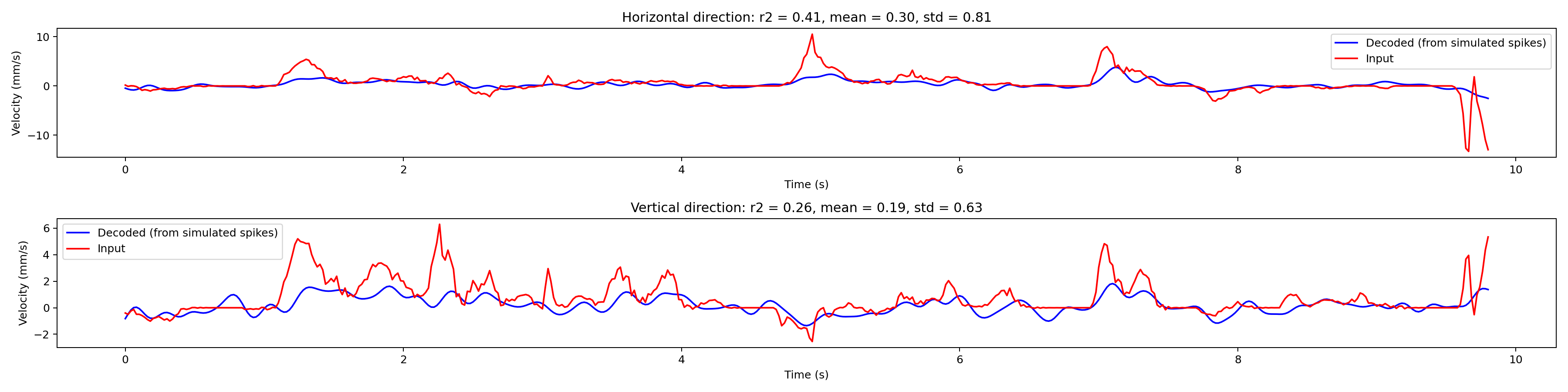
Total running time of the script: ( 0 minutes 0.561 seconds)